Predict
Case study Blackboard
I co-led the user experience design across both web and mobile platforms for Blackboard's student advising product, which provides academic advisors and instructors insight into student risk analytics and outreach tools to better support student success.
Experience & Product Designer
2016 – 2018
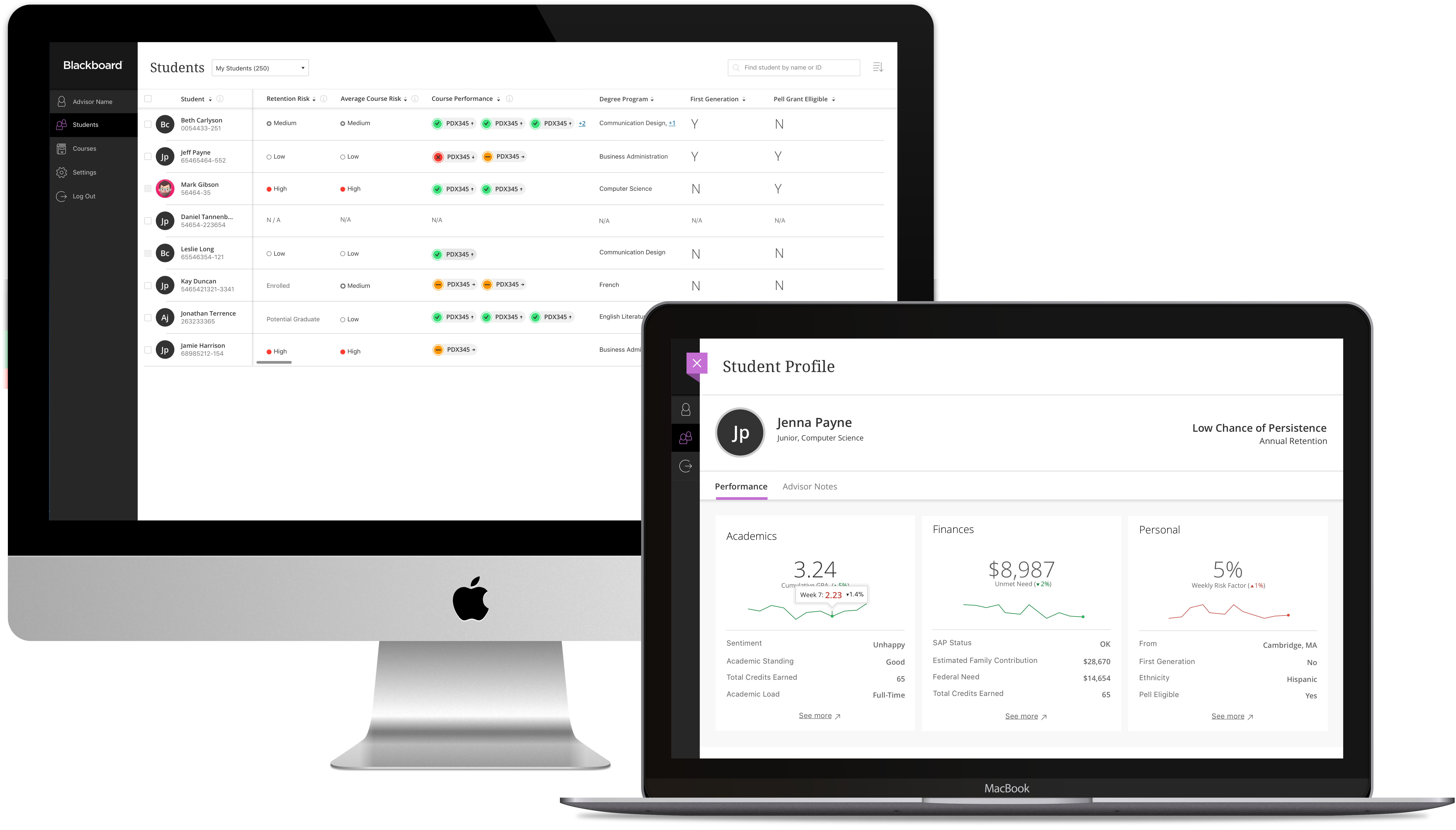
Problem
With large class sizes, growing advisor to student ratios, and siloed data accumulation, staying informed about student performance is difficult, particularly in the absence of real time data. Advisors and instructors need tools that provide insight and intervention opportunities to help students overcome barriers to academic achievement.
Goal
To help advisors and instructors leverage data and advanced analytics to identify students at risk, making early intervention possible, personal, and scalable. Increase retention and graduation through intensive advising on the basis of early alerts.
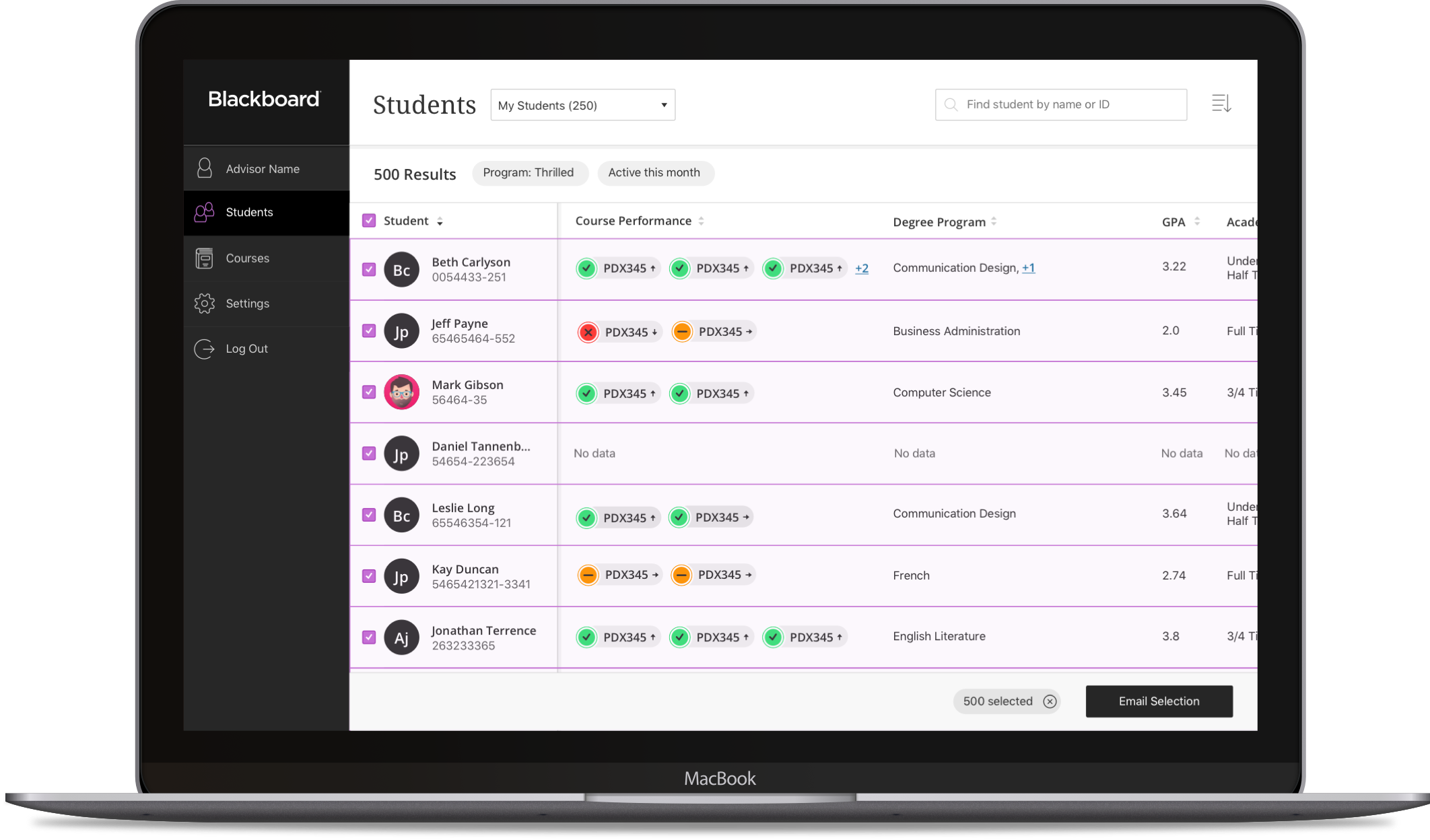
Skills
Interface Design Interaction Design Responsive Design Accessibility Design Wireframing Usability Testing Visual Design
____
Sketch
InVision
Zeplin
Team
Dan Shipley
Jun 2016 – Dec 2017
Overview
I teamed up with another designer to build the following core features: a
documentation platform that allows advisors and instructors the ability to
share their notations on student performance; batch email for advisors to scale their communication reach; and a redesign of the student profile to reflect a new predictive model focused on student retention analytics.
Working within an established design language system, I designed new components, archetypes, and color palettes.
Working within an established design language system, I designed new components, archetypes, and color palettes.
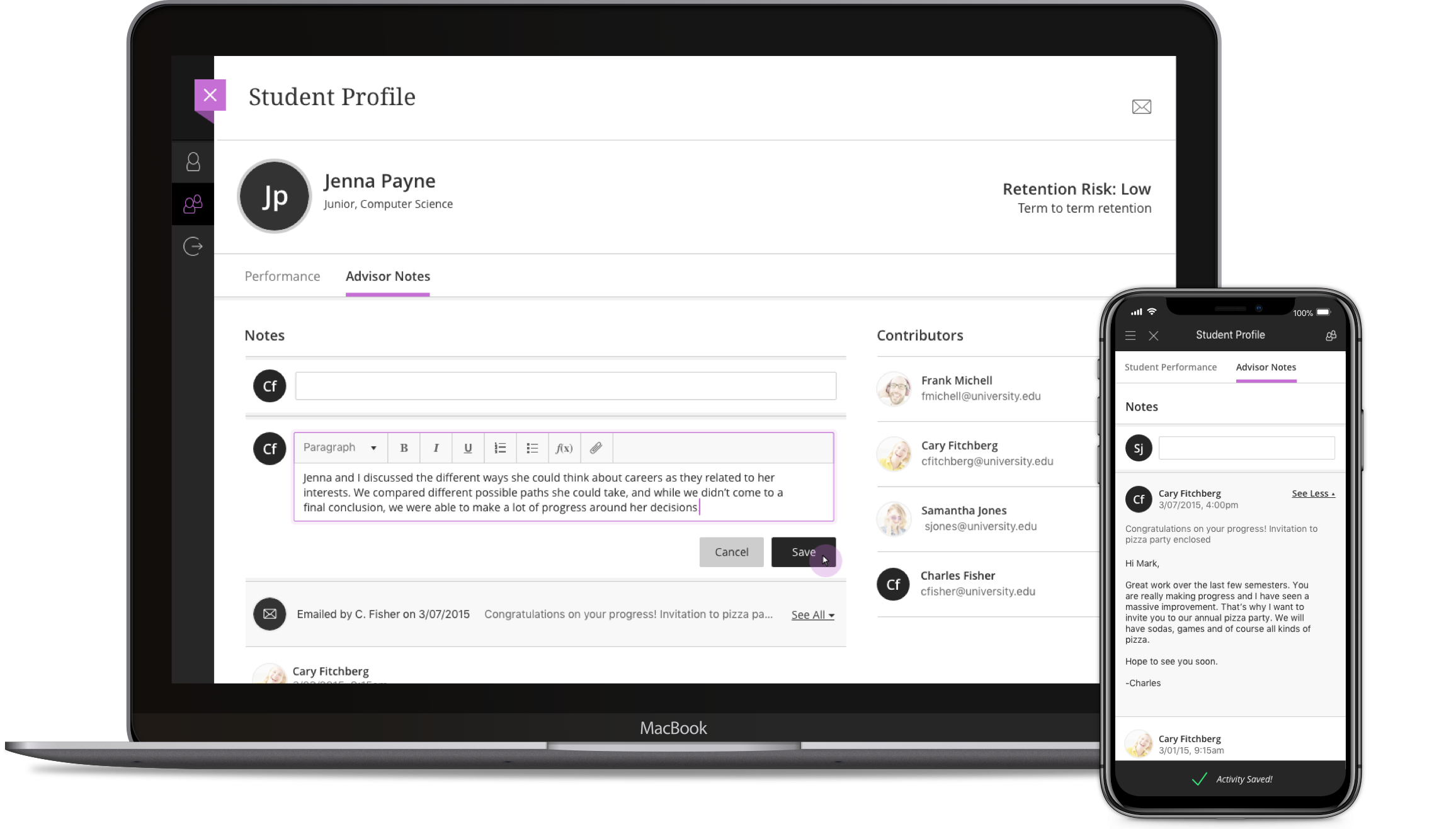
Shared Advisor notes
Advisors often carry caseloads of hundreds of students and keeping records about the progress (or regression) of students within the context of real time performance data can be difficult.
Here, we've enabled advisors to document their communications, interventions and student follow-ups.
This also enables visibility into previous conversations for future advisors.
Batch Email
Advisors want ways to scale their communications so they can improve the situations of more students at a faster pace. Batch email helps advisors scale interventions by emailing entire groups using built-in filters.
New Predictive Model
What is the probability that a student will return the following semester or year? Part of contributing to student success is reaching the right students at the right time. A new predictive model was created to help identify those students most at-risk for attrition in the next term for proactive outreach and intervention.
Users will be able to triage, sort, and filter students by retention risk; have insights into additional factors known to influence retention risk; and have the ability to dig deeper into the academic performance of students at high risk of attrition using existing course-based predictions.
We needed to understand what data would be available, what that data would mean to advisors, instructors, and students, and then reconfigure that data and present it in helpful and intuitive ways for users.
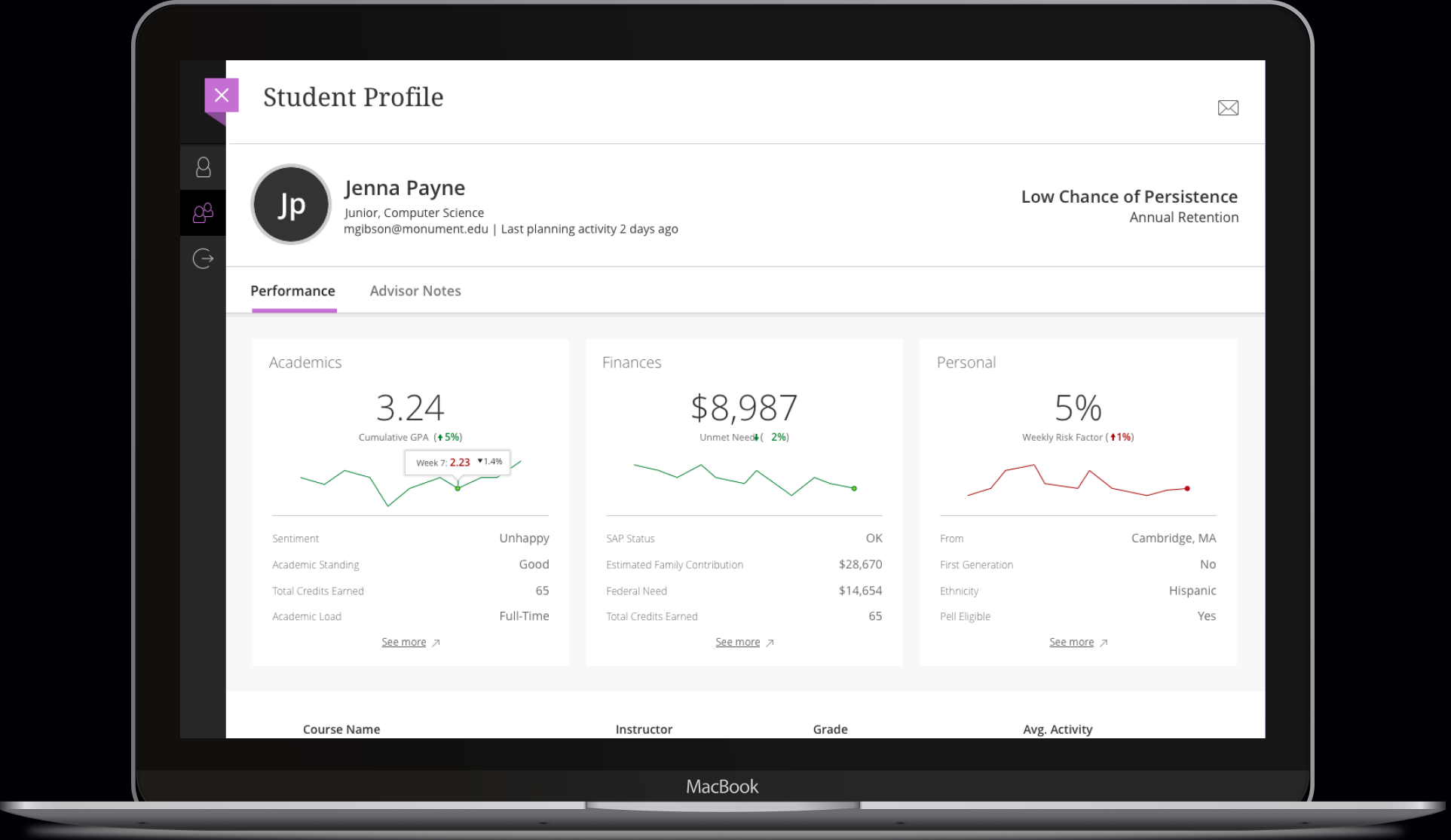
MVP
Because the data model was in a nascent stage, we couldn't yet determine the extent of its potential and therefore had to design a foundation for the model that could also adapt to its growth.
In working closely with the data science team, we designed an MVP version of the data model, exposing only the information we knew we had: a student's chance of persistence based on course performance.
Post-MVP
As the data model grows from greater participation in analysis and access to institutional data sets, the student profile will evolve to function more as a dashboard, having customizable data visualizations illustrating the factors behind the prediction.
The dashboard is intended to be configured by users so they can determine what information is most important for them.
Wireframing and iteration
When we started working on this product, a product strategy roadmap had already been established. We were tasked with building core features with the understanding that advisors were intervening when it was already too late.
However, after our first iteration of ideas, we realized we were working on assumptions of the advising space without having any first-hand experience or support.
In order to better meet users' needs, we interviewed advisors across the country asking what their experiences were tracking student performance and what tools might allow them to more effectively contribute to student success. Here were some of the questions we asked:
Q:
What are the key items you need to know about a student before meeting with them?Q:
How do you currently know if a student is at risk of poor performance or attrition? Q:
When was the last time you intervened in a student's academic career and how did you do it?Wireframing
Next, I created low fidelity mocks-ups of all the key flows. First, I identified each key feature and how it would function within the information architecture, and then I built each feature out flow by flow.
By creating wireframes without color or fanfare, I was able to work quickly and communicate the idea without potentially distracting visual components. Additionally, working in this way allowed me to coordinate common interactions between the key features and design them cohesively within the system.
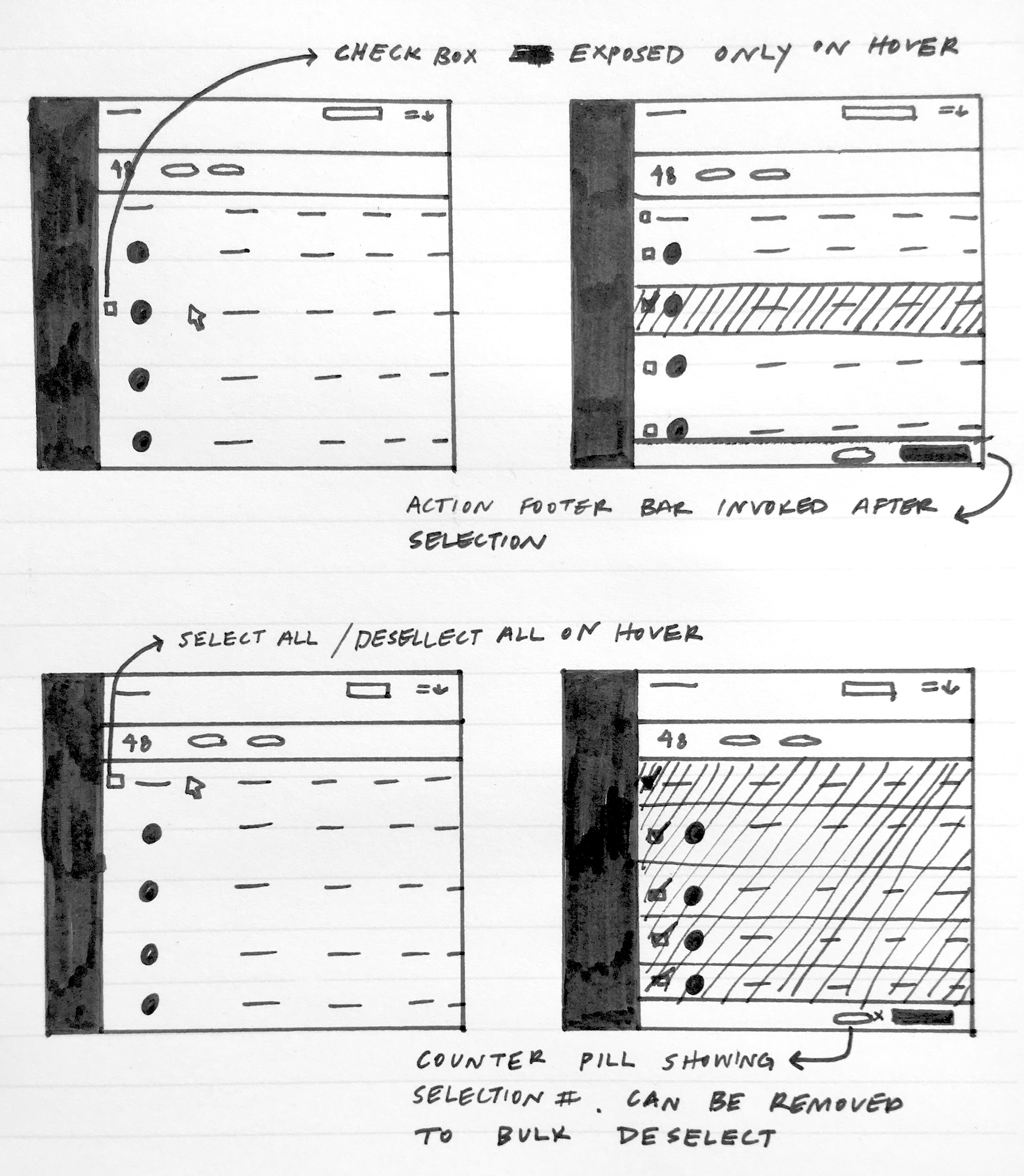
Higher fidelity wireframes
After identifying the key flows and interactions, I created higher fidelity mock-ups and identified any unique components or archetypes that would be added to our design language system. I then worked with the product owner to identify any edge cases that would need to be accounted for, building out each scenario flow by flow.
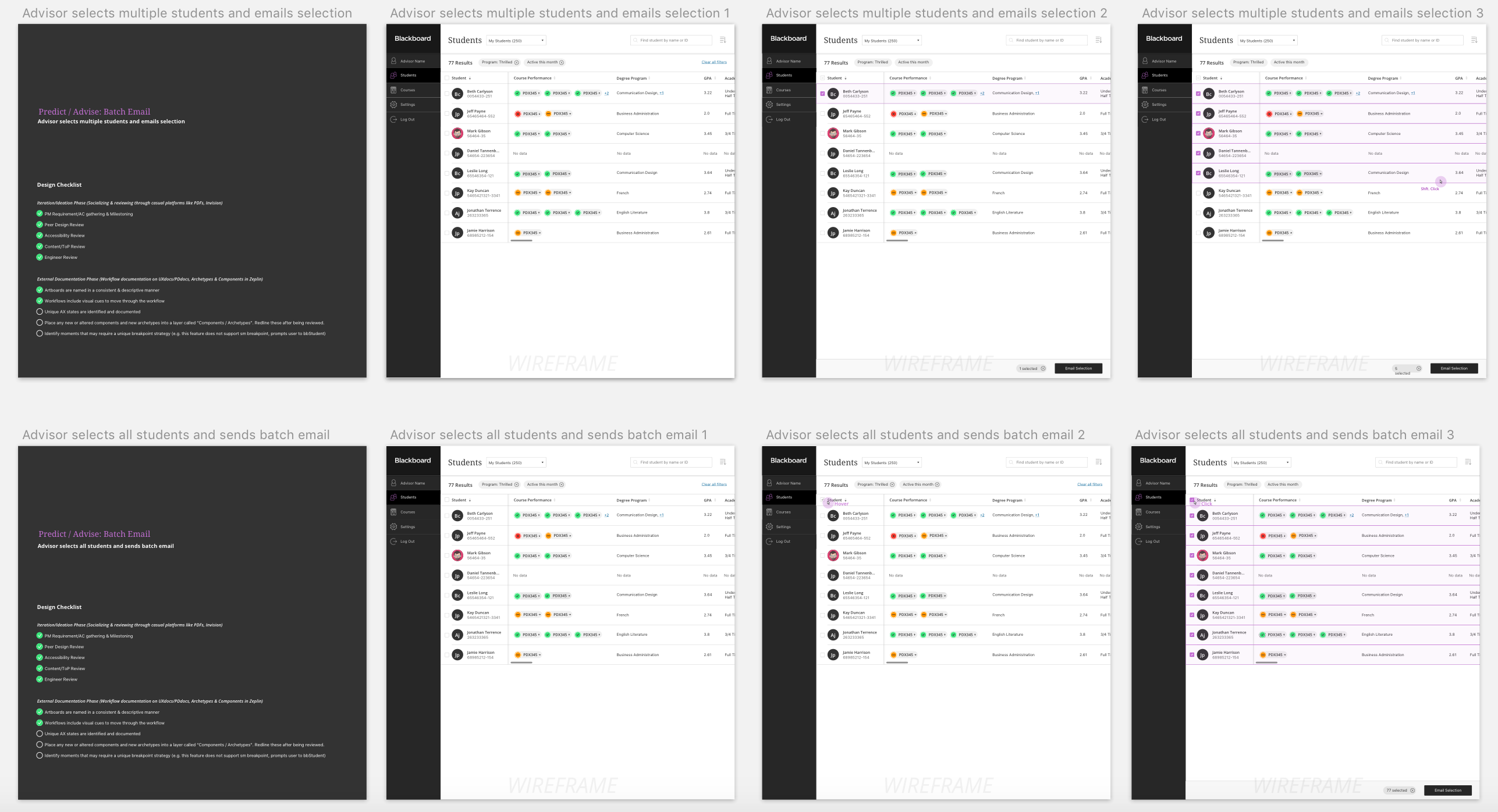
Rapid prototyping
After building out the flows in Sketch, I linked them together to create prototypes using InVision. This was essential to make sure I wasn't missing a step in the flow. It also helped me share the concept with the product owner as well as the developers that would build the app.
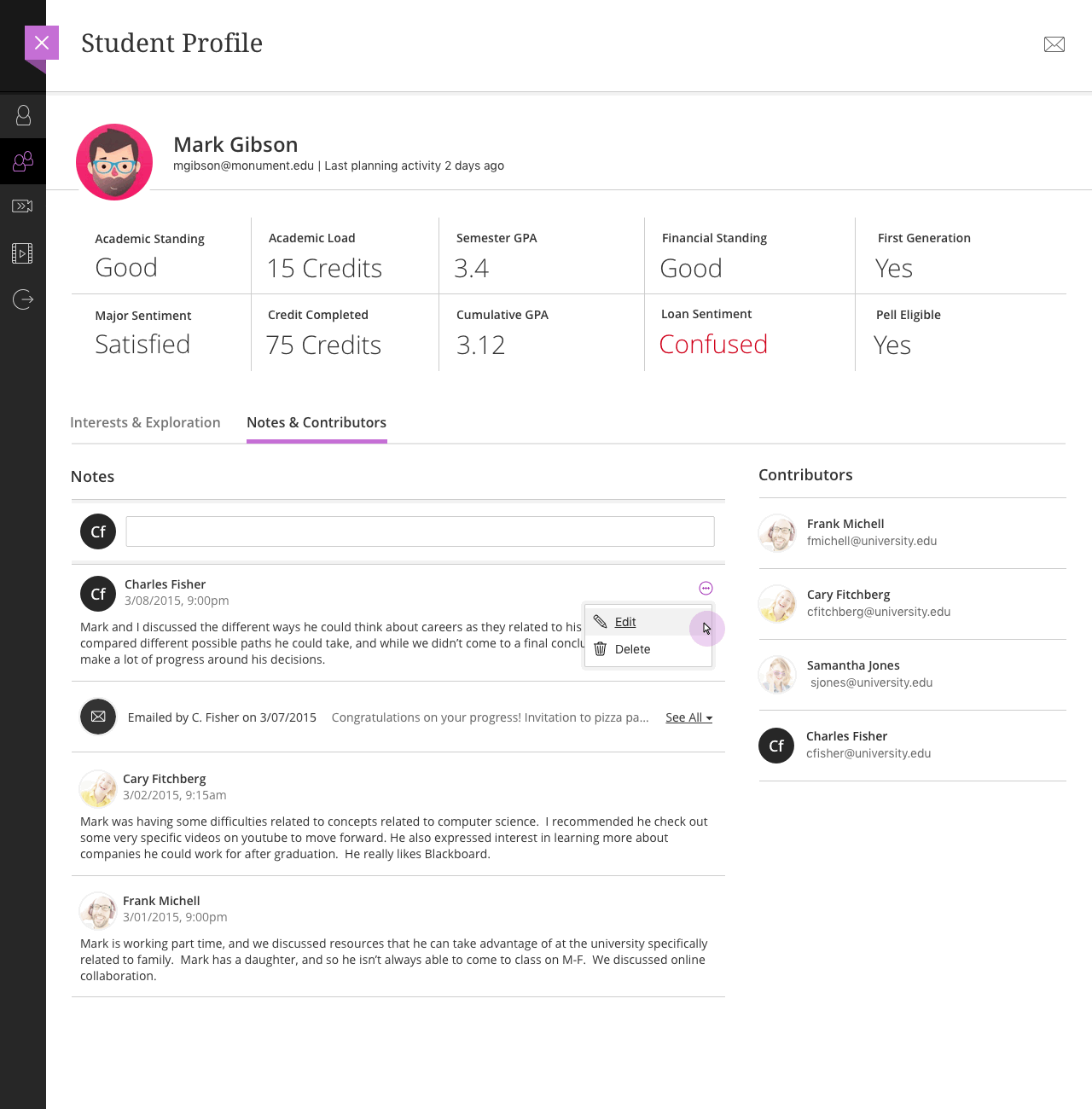
Usability Testing
Next, I wanted to understand how real users would think and interact with the interface as they moved through each flow. Where were the biggest hang-ups? We set up interviews with local advisors and instructors and conducted usability tests using the Think Aloud Protocol. We went through two rounds of testing and iterating on the key flows.
We also held client meetings and conducted evaluative research to review and iterate on current designs and future concepts to keep pace with our tight schedule and meet our strict deadline.
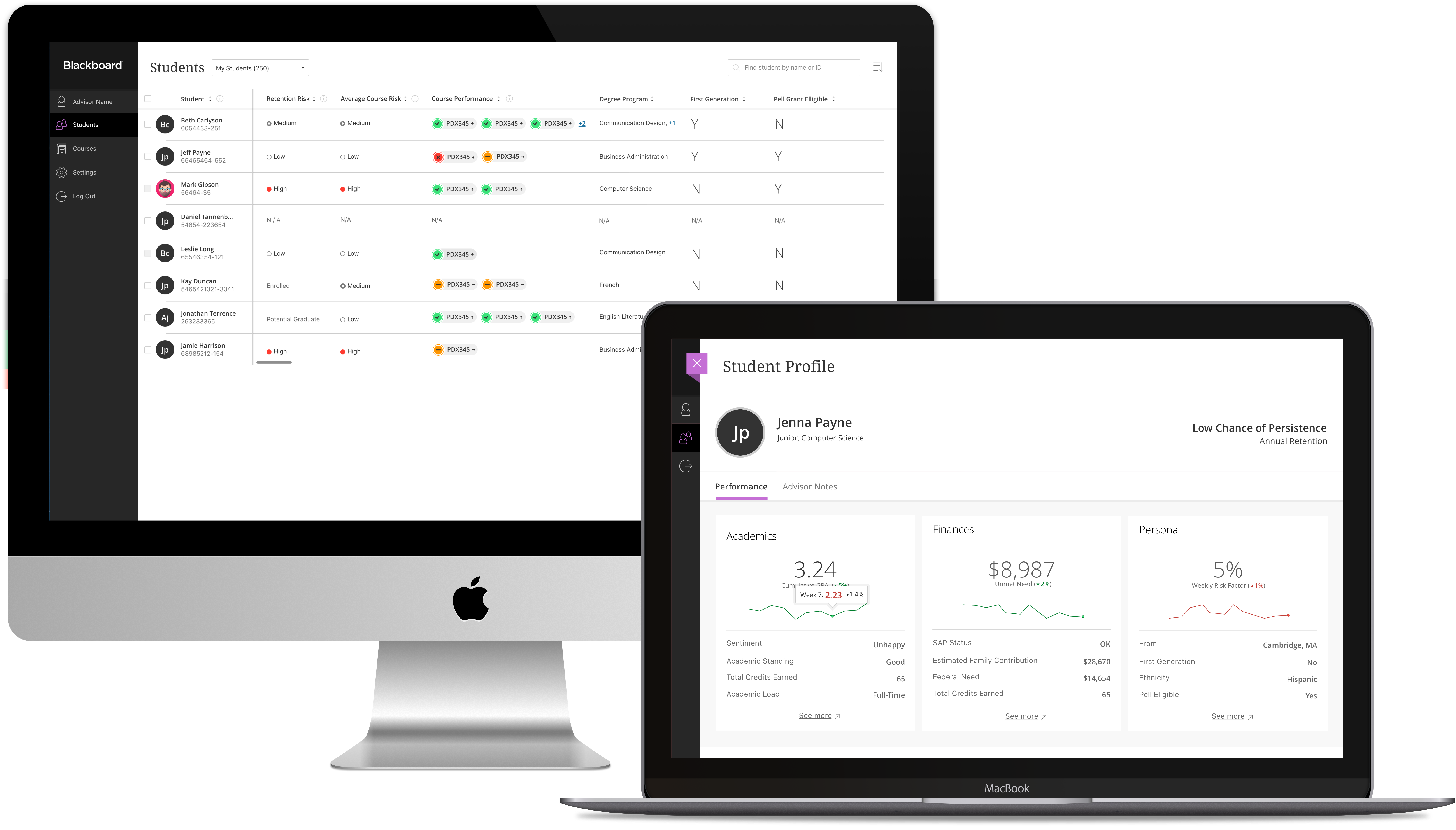
Development and sizing
Once validating and finalizing designs, I met with the development team to size out all the features and visualize a product roadmap, providing redlined high-fidelity wireframes to engineers and product management. I carried out these designs through development working with the product owner, scrum master, and front-end developers.
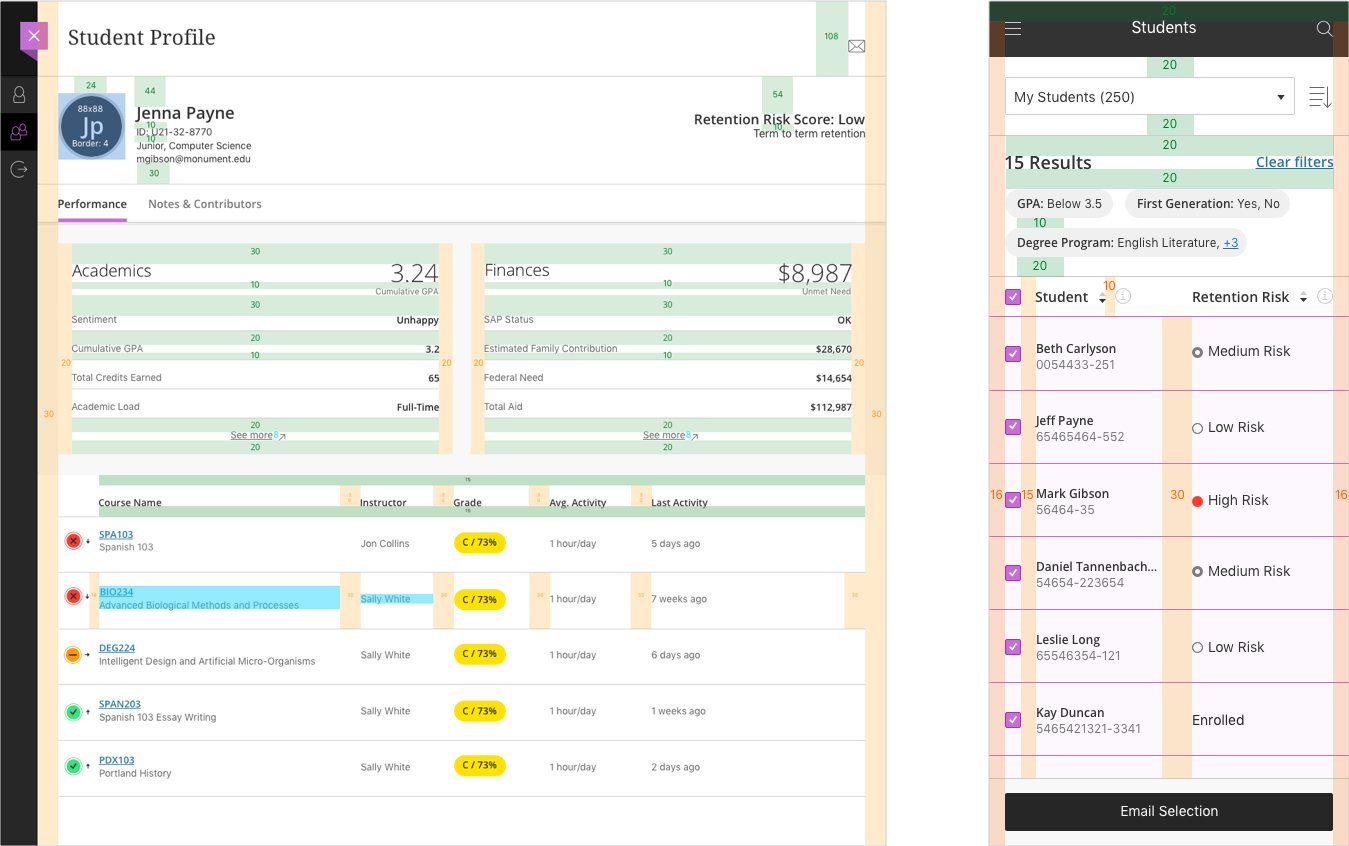